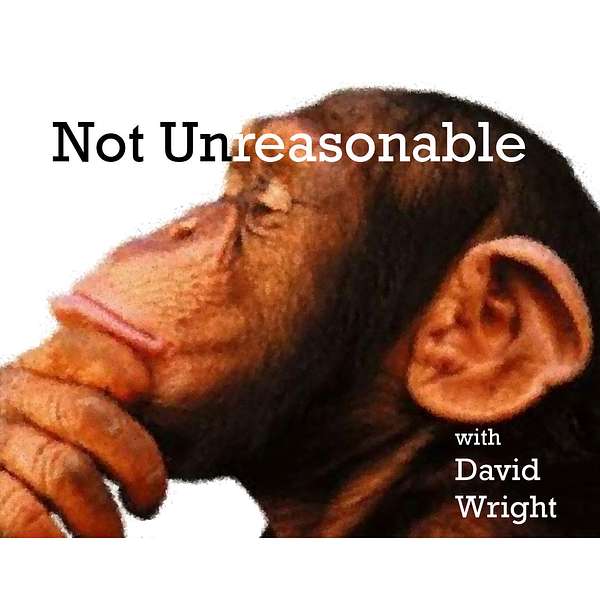
The Not Unreasonable Podcast
Subscribe in iTunes, stitcher, or by rss feed. Sign up for my newsletter here and also see us on youtube!
Show notes at notunreasonable.com
The Not Unreasonable Podcast
Doug Hubbard on How to Measure Anything
Doug Hubbard is the author of several books and I've read two: *How to Measure Anything* and *The Failure of Risk Management*. I can honestly say that one of my career goals is to implement his methodology into my job today and everything I do in the future. Here's an incomplete list of wow realizations that I had reading Doug:
That you can overcome cognitive bias in estimating variation
That we don't measure what's most important
That we can quantify the value of information
That we can quantify uncertainty and use it to make decisions
That expert opinion can be calibrated and aggregated and use in a quantifiable manner
That Bayesian statistics explain the reduction in uncertainty that accompanies additional information.
That last one is an *empirical* observation. I remain floored by that. Floored.
I didn't even cover half of what I wanted to cover with Doug. Read Doug Hubbard. Learn from Doug Hubbard. I will continue to!
Show notes:
https://notunreasonable.com/?p=7298
Twitter: @davecwright
Surprise, It's Insurance mailing list
Linkedin
Social Science of Insurance Essays
My guest today is Doug Hubbard of Hubbard decision research. Doug is the author of several books, among them how to measure anything funding the value of intangibles in business, the failure of risk management, why it's broken and how to fix it. Doug, develop a fascinating framework called applied information economics that we'll be digging into day to today. Doug, welcome to the show.
Doug Hubbard:Yeah, thanks for having me.
David Wright:So we're gonna be digging into and celebrating AIE applied information economics today, which is a very rich set of ideas. And I see this kind of, generally speaking as how to make people make vastly better decisions and conditions of uncertainty. One of the things that occurred to me though, as I was reading the reading through all your material is that a lot of big decisions are made by organizations, which, of course, has an individual decision maker, which we can touch on, as well. But organizations tend to process information to make decisions, using other means than just Hey, is this a technically correct thing in the mind of one person, their political decisions? And in some ways, I think, a framework, a new framework, and unfamiliar a framework for ideas within an organizational decision making process can almost be seen as a threat, right, where, you know, you have this new information, new way of making decisions. And I think that the way in which that would present itself to you probably is that is a consulting project where you would recommend a decision or they would recommend a decision with your help, we just get killed silently, and just wouldn't get made, even though it seems obvious that it should be made, and you have no outcome. In the end, I'm wondering if you haven't experienced this kind of like irrational resistance from an organization to to to your work?
Doug Hubbard:Well, you know, I've I've had several federal government projects. And so there's a whole other set of reasons why sometimes they follow through with a recommendation or don't. But actually, I find it useful information by itself. I, one of my earliest clients was actually an insurance company that was going through a merger. And I had done a risk return analysis on the development of this major new piece of software for the organization and the in the risk analysis. And we measured benefits and all this sort of other the cost and the duration and the things that might happen during an after a merger. And we ended up saying, look, there's just too much risk before the merger, you should wait until after the merger, because the we've looked at the data and the chance of cancellation of major projects after mergers is pretty high. So why not defer this? So after all of this analysis, we said, well, your main risk is just that after a merger, when they consolidate systems, they end up canceling this. And they said, Well, thank you very much, but we're going to go ahead with this anyway. And then, of course, after the merger, the project was cancelled. And they had spent an extra few million dollars between the time I made that recommendation, and the time was actually cancelled. So in some ways, I see it as an opportunity. People always ask, but how would you know what would have happened? Otherwise, if they didn't follow your recommendation? Well, we have a few data points on that. So we have some situations where people did something otherwise, we try to take advantage of that actually, and say, What did happen after they didn't follow our recommendations? It seems more often than not, what's interesting, is that they go ahead with a large project that we recommend that against as opposed to the other way around. Sometimes we end up recommending they do something that they end up not doing. But actually it's the the former more often, it seems we do some analysis on a big investment initiative could be civil engineering, engineering projects, could be software projects, new technology r&d, and we say don't do it or defer it or wait until these conditions arise. They go ahead and do it.
David Wright:So what is what is the default model they're using? Do you think? Why do they make that is what is what is driving that decision?
Doug Hubbard:Well, I think in some cases, frankly, it's an I should state that most of the time clients actually follow our recommendations.
David Wright:But well, that's why they brought you in was for your recommendation.
Doug Hubbard:They think that they were there, but they actually do have a dilemma. They don't know what the best strategy might be. They have difficulty measuring things they want to know. And these are the people making the decisions or writing the checks, and they would like to reduce their uncertainty and make a better bet. So those are the clients that are more likely to follow through with actual decisions. But sometimes in all management consultants know this, a client brings you in because they kind of made up their mind already. And they're pretty sure that a consultant will agree with them.
David Wright:Okay. And they want to they want to bolster their case.
Doug Hubbard:Yeah. And they're surprised sometimes in the findings that the consultant does not agree with them. So I've I've always said, Look, my Customers are the ones that actually want to know the answer. You know, like, there's a lot of firms you're gonna hire if you just want someone to do agree with you. But if they actually want to make better bets, make better decisions and serve their customers, their investors, their employees better. The general public, if you're a government agency, if you want to serve them better, you want to, you know, have less uncertainty in your decisions in strategic ways.
David Wright:So there's a idea I want to get right on the table right away, which is, I mean, in a, in a, in a list of profound ideas that you've taught me through your work, this one maybe stands at the top, which is the idea of risk aversion. And risk aversion is this observation, which is that the riskiest things are usually the ones that you don't measure. Oh, yeah.
Doug Hubbard:Measurement inversion, right?
David Wright:Measurement inversion. Yes.
Doug Hubbard:Yeah, the the measurement inversion, the I was expanding on decision analysis, which came from Ron Howard at Stanford. And that was an offshoot of decision theory, Game Theory sorts of stuff. So the whole idea of computing the value of information before you make decisions, they were applying it and pretty straightforward in their, in the academic examples they were using, it was pretty straightforward, simple decision tree stuff. But in real world decisions, you know, people are making cost benefit analysis models in a spreadsheet, where there's a big number of costs and benefits and a big cash flow over time. And there's a large number of uncertain variables that are continuous values. So you have to describe your uncertainty on as your actuarial listeners will know, you describe their uncertainty with a probability distribution over the possible values of a particular quantity. And how you compute information values on something like that is a little bit more complicated. So we started systematically applying that. So we took a decision analysis, we combined it with the idea that a lot of things that people say are measurable really are if you think about it from a Bayesian statistics point of view, and think about measurement as a quantitatively expressed reduction in uncertainty, not necessarily elimination. And a lot of research on decision psychology, which methods actually measure measurably outperform others, and so forth. We put all this together. And we started applying these decision analysis methods of large decision models with lots of cost benefit variables over a long period of time, lots of uncertainties, and we started systematically computing information values on each individual variable. And just for your listeners who might not be familiar with it, the value of information comes down to making a better bet, if you had less uncertainty, you're more likely to make a better bet, there's a way to compute the economic value of that. Well, when we did this, for a large number of these decision models, and these decision models would be cost benefit analysis for some initiatives, software, public policy, or something that might have a couple of dozen, or maybe a couple of 100 variables. And we would compute the value of information for each individual variable. And what we found out is that the high information value variables are often not what the client would have measured, otherwise, they would have measured something differently. And in fact, if you look at their past behavior, you tend to find out that the things that they spend the most time measuring are the things that actually are statistically less likely to improve a decision. And the highest information value on average, were often things that were just completely ignored. They they're not even in the business case, much less measured. So
David Wright:amazing. I mean, it's it that I think it's worth just dwelling on this for a second longer, because, you know, I listened to, you know, a few, a few podcasts of yours that you've done. And, you know, it's, this is big, deep, important stuff, you measure the things that are easy to measure, that are just laying around in front of you, because you can measure just by not lifting a finger, but they're not the things that matter. And you and I want to make another kind of really important point here about your work, which is that your your your assertions and your conclusions, these aren't things you're pulling out of the air. I mean, it's a very, it's a it's a thread that just throughout all of all, everything I've read of yours, which is it is all grounded in research and empirical analysis. So when you say the value of information, what you mean, is you mean that we have computed the risk without the information, and then the risk with the information. And we've we've calculated the difference in value between these two scenarios. And that is the value of information very profound.
Doug Hubbard:Yeah. Well, thank you. And actually, that's an important point to talk about the precision of the language here, because there's a lot of fluffy stuff out there. I was just having a discussion on LinkedIn, actually about the coso framework. You mentioned that, you know, a lot of your listeners are actuaries. But they might not be familiar with some of these MRM frameworks out there. There's these kind of soft management consulting kind of auditor driven frameworks. And they come up with standards. They look structured and formal, but they tend to resort to qualitative methods, like a very simple risk matrix. And if some of your listeners aren't familiar with that, you can just Google that and see a picture of risk matrix. It's got red, yellow, green squares on and so forth. And this apparently constitutes risk analysis. for about half of organizations that are practicing ers, we've actually done surveys in cybersecurity, risk, project risk and enterprise risk management. And the slight majority in all of those is somebody using this qualitative risk matrix, only about 20% are using quantitative methods for risk analysis. And I remember years ago, telling actuaries that some in some cases, I was interviewing actuaries for certain parts of a couple of first books. And I would tell them about these methods. And they were surprised to hear about them, they did not know about them. And they were surprised anybody would ever do any risk analysis that way. I said, No, I'm not kidding. This is actually what they do. And they make these big decisions based on that. So these aren't trivial decisions. These are big corporate strategic decisions. In fact, I think the main reason that these qualitative methods might not do more damage than they could is, I think people intuitively and probably correctly override these results, they ignore it. Or they gain such a way that it kind of matches their intuition anyway. So it probably, it probably ends up being in the category of what I would call merely useless. Yes, other researchers, I cite Tony Cox's work there, where he did a lot of really a method of quantitative analysis of risk matrices. And he concluded that many of them can be worse than useless, which was not just a waste of time, but they actually added error to the expert intuition. They diluted the expert knowledge. They added ambiguity to the process. So they actually made things worse, they create things that other researchers had called the illusion of communication. You and I both agree that a risk is medium. We feel like we've accomplished something because we agreed on it. But it turns out, we are completely different things by that, I'd say there's researchers I cite on that particular front.
David Wright:So you actually deliver a pretty interesting critique of ordinal statistics, right. And there's a there's a variety. Typically, I think a lot of these risk matrices, they tend to sort of have this, you know, a scale of one to five, or you say low, medium, high, that kind of thing, right? But these ordinal statistics are actually pretty horrific. And it's not numerical analysis. It's not quantitative analysis, right. It's something entirely different, has its own
Doug Hubbard:pseudo math. It feels like math to people. And here's a really important thing. This is a really important question for actuaries and all quantitative analysts, or analysts of any kind to ask, how do we know it works? Even the mathematical methods? How do we know it works? Well, the thing I was going to point out before was, there's actually been some really large clinical trials, some of them with more data than the COVID vaccine test. Philip Tetlock did the 20 year long, good judgment project, he tried to 184 experts at 2000 individual forecasts he tracked and he concluded there, he couldn't find any domain in which humans clearly outperformed crude extrapolation algorithms, naive statistical algorithms, but but based on historical data, and so forth, not these ordinal scales. And so I spent a lot of time citing a lot of research about these ordinal scales and so forth. And the problem with risk analysis and risk management in general is it's not an area where analysts and decision makers can get immediate and ambiguous feedback. So if you said I did something that reduced the risk of let's say, a cybersecurity data breach from 10% annually to 5%, annually. How many years? Would you have to wait to observe that that was the case? And did you even know that the 10% was correct in the first place, you're not going to get immediate feedback and it won't be unambiguous. It will be highly delayed feedback, assuming you're tracking it at all. Most people aren't even tracking it. in that kind of environment. Experience does not turn into learning. Daniel Kahneman and Gary Klein actually did some research on this and they found out they agreed on this one thing they kind of came from different areas. Daniel Kahneman won the Nobel Prize in economics in 2000. To develop behavioral economics was the basis for behavioral economics of what he called Prospect Theory and so forth. in these areas, one of the things they agreed on is you had to have a consistent, immediate unambiguous feedback for experience to turn in for learning, and we just don't have that in risk analysis. So here's the issue. How can we know what works? Well, we can't rely on our impressions of what works, because there's another set of research that uses the term algorithm aversion. And there's also a set of research that I've dubbed analysis placebos, these aren't the terms that the authors used that second set analysis placebo, I did group them together on that, because they're all saying the same thing. The problem with analysis placebos is that you can easily adopt a method that seems structured and formal. And your confidence will inevitably go up, even if the method improves nothing, or even makes things worse, for your estimates and decisions. It's almost inevitable that your confidence will improve if you're working on something that doesn't give immediate feedback. But it seems structured formal, it seems like it should work, right? And you just feel better about your estimates and decisions. So there's a site multiple studies that come to the same conclusion from completely different areas. And then I said, these are all kind of saying the same thing. I'm going to call these analysis placebos, we can't count on our impression, our perception of effectiveness of a methodology that has highly delayed and consistent, ambiguous feedback. That's why when you do vaccine trials and clinical drug trials, you have to have control groups. They can't, you can't just assume that, well, if people are feeling better, they must actually be getting better, right? Or because the doctors think the drug should work that it will work, right. That's not how we test drugs or vaccines. And we shouldn't test our risk analysis methods that way. And we have science. And another really important thing is that when it comes to perception, apparently, algorithms are swimming upstream a little bit. And that's because of this effect called algorithm aversion there is a tendency to grade algorithms more harshly for errors than they would humans. Yes. So even if decision makers, they tested this in clinical trials, as well as experiments, even if you give people who are trying to estimate things, the actual records of past errors have algorithms to forecast something versus say, human experts or their own estimates. If the algorithms make almost any errors at all, they'll tend to default to the human errors, people starting with the human, they'll accept much bigger errors from the human before they ever consider switching over to the less erroneous algorithm. So if yours that the decision model they're using is this method has to be nearly perfect. If it's not, I default to a clearly more imperfect method. And that's a that's a problem. So how do we go about measuring the effectiveness of risk analysis methods with this feedback prop? Well, there are methods. One is we can do what we call component testing. All right, so software engineers, and engineers, in general familiar with this concept, lots of the components of risk analysis methods have been studied thoroughly. Like for example, the all the research on ordinal scales, we know something about the behavior people have with ordinal scales, we know something about the behavior people have with putting two ordinal scales on a matrix and putting dots on it. We've measured something about the ambiguity of the terminology, and the unintended consequences of arbitrary features of the scale, right, you arbitrary features of the method actually have a huge impact on how people use them in a way that the designers of the method really didn't anticipate they will be capable of that.
David Wright:Can you elaborate on that a second, what would be an example of a design that would would influence behavior?
Doug Hubbard:Oh, sure. So you would think, for example, that if I had a five point scale, and I decided to go from a five point scale to a seven or 10 point scale, then I could kind of extrapolate people's distributions. Imagine i'd measured the, you know, a little distribution of how people how often people chose a one on a scale or a two on a scale. So I've got popular this distribution on this scale. And I extrapolate that to a 10 point scale, I decided to go to a 10 point scale, you would think that you could kind of extrapolate
David Wright:that the shape of the distribution would be the same.
Doug Hubbard:Now Yeah, it turns out, that's not the case. There's this one really weird effect called partition dependence. This guy right from last was studying this. Suppose I want at a five point scale, where they're measuring impact, right? So they measure impact sometimes in dollar values, let's say and a one means less than a million dollar impact. Maybe that's what a one means. And then you've got this five point scale and you got you defined to mean greater than a million, but less than 5 million or something like that up to five. All right, maybe five means 20 million plus or something. Well, suppose you went from that to a 10 point scale. And of course, you have finer and more buckets. But maybe the first one is exactly the same as defined exactly the same as the one on the five point scale. So both of these ones are defined as less than a million dollar impact. Why do people choose one less frequently on the 10 point scale than they do to a five point scale? You defined it exactly the same? Well, we mark this subjective algorithm to want to spread out our answers, right. So it turns out that people don't really pay that much attention to the definitions that people that the designers laboriously tried to come up with, right, they came up with all these detailed definitions of what one means and what a two means, etc. And they may be influenced by that, but they appear to be influenced just as much by the subjective tendency to spread their answers out.
David Wright:That's incredible. Okay, I like to just sort of make just sort of pull out two points to kind of themes here that have been introduced, I'm gonna keep going on these themes. So just to make sure that we talked about them. So on the one hand, you have this observation that people that the decision making process that organizations go through, or people go through, do not appear to actually work, right, in the sense that they don't, they don't drive better decisions. And the other one you have the design of any particular system will introduce biases, right. Which, you know, can cause the system to fail. But on the first one, I just want to kind of run an idea past you, which is that, you know, back to the kind of the original question, which is, it feels to me, like in a lot of cases, the thing that organizations want is actually consensus built. They don't necessarily want decision. That makes sense, right? So they want you whenever you're on the same page, and in that sense, you know, these kind of random analytical framework, which gives us confidence that we're being scientism, right, so we're like, looks like we're doing science. And so we all feel good about that. And therefore we feel good about, you know, developing a consensus to take a particular action. Like, I think of the difference between the kind of like an executive in a company and a more junior person in a company, the executive spends all their time building consensus, right around taking actions in the organization. So that's actually the hard thing to do in that model of an organization. And anything that Beltsville consensus is a beneficial tool for an organization. That doesn't mean they're making good decisions, that's going to be right, it can be random.
Doug Hubbard:I'll go even a little bit further. Because consensus is part of another objective. I think a broader objective might be just anxiety reduction. Okay. So whether they have to build consensus with a team or whether they're really just making a decision for themselves, what they want is less anxiety about the decision. Right? That's another way to look at it. And that includes the consensus building objective. So that I would say, Well, if that really is your objective, I wouldn't refute that. I wouldn't argue that, you know, there are plenty of ways if if you want to build consensus, or reduce anxiety at any cost, then sure, luck, these methods could work. I think some people though, at least, sometimes actually do care about making better bets, that sort of thing, especially when they include the allocation of large resources, and in many cases, even public health and safety. Right. You know, the returns on investments for, you know, investors in a large corporation, or the satisfaction of your customers, these things matter. If, if that's what you care about that. And and I'm not going to try to say they should have a different objective, I would say, look, if your objective really is just anxiety reduction, I guess that's the way to go. But I think, and I hope a lot of my customers are actually trying to improve their decisions. They're actually they actually do have a dilemma. They're not sure what to do. They want to try to reduce the chance of big opportunity losses by either rejecting what would have been a good investment or accepting a bad one, right? They actually do want to reduce the risk on those sorts of fronts. when that's the case, well, then all the evidence says you really ought to be using more quantitative methods. There's even more so than the evidence against the qualitative methods. There's plenty of evidence for quantitative methods. Paul Mele, a researcher who had been comparing human experts to statistical models since the 1950s, all the way up until he died in the early 2000s. Here, he'd collected over 150 studies, he said he could only find six cases where the humans did slightly better Or just as well as the algorithms, that's incredibly clear for the algorithms.
David Wright:That kind of so I want to, I'm going to try and tell a more sympathetic story, if I can, and see if see if we think about this. So in a certain model of an organization's decision making process, if you have enough diversity within the decision making group, right, you're going to have enough expertise that's going to, if you can get consensus among a diverse enough group, then you probably have considered a lot of things that no individual can think of on their own. Right, and what there's one of the themes about your work, which I find interesting, we're gonna come into the AI framework in a sec, we're just gonna gonna really pull this point out, which is that, you know, real expertise is a very important source of information, right? If you don't know what to do, the first thing you should do is check the research, or ask somebody who really knows what they're talking about, and they will be able to help you, right. And if you can integrate a lot of different sources of expertise. And I think large organizations have a lot of different sources of expertise, then you could argue that they're actually not doing too bad of a job. They're kind of coming, you're sort of falling backwards into a methodology that is not explicit, but but implicitly doing the things that that that you would you would agree with, even though they're sort of not nobody's necessarily conscious that that's what's happening. But they do every day is these executives, as they spend their time trying to create consensus, and the process by which consensus is created is actually getting diverse views into the pot to consider things properly. But it's very messy. Right. And I think that's kind of problem that you're not actually being explicit about what you're considering. You're just sort of making your discernment. And you can you can crop that process through like a charismatic leader or whatever, can just sort of railroad something through, you know, like it's just unscientific.
Doug Hubbard:Sure.
David Wright:What do you think about that?
Doug Hubbard:And that that kind of gets back to that illusion of communication. I talked
David Wright:Yes, right. Exactly. Yes.
Doug Hubbard:It people, it may only appear that there that there's consensus. So two researchers desk who looked into this quite a lot. And this is where they found that even the verbal labels that people use for things like likelihood and impact and risk where they say this is unlikely, or medium versus moderate, versus this is critical, or extreme or extremely unlikely, or something like this. Those arbitrary choices of the words you use actually also have an impact on people's distribution of responses. And it also leads to that illusion of communication thing again, which is You and I both agree that something's unlikely or medium risk or something. And we think we agree, and in fact, we don't. So yes, those are all good points. I would say this, when it comes to methods for aggregating multiple experts, that's a testable hypothesis. Yes, good news is there are methods that have been tested after I just got done talking about the, you know, relative performance of algorithms over subject matter experts. I will also say there are ways to use subject matter experts that measurably outperform other ways of using them. So in the AIA method, we don't reject the use of experts. What we say is just do less of the math in your head. Make the math explicit. Okay, make sure you define your terms, well get the math out of your head. And where you do need to rely on human experts, there are ways to use the human experts that measurably outperform others we know that experts, for example, are systemically overconfident when they estimate things, and you can adjust for this sometimes researchers call this D biasing you can adjust for this overconfidence bias through training, we've actually calibrated it's called calibrated probability assessment training. We've calibrated over 1600 people in the last 22 years. So we have a lot of data on this. What does nobody
David Wright:tell us what you do when you do that is an amazing idea.
Doug Hubbard:Yeah, calibration is just the idea that if you give people practice, they can get pretty good at putting subjective probabilities on things. It's a skill you can learn, right? Just like you might not normally need to estimate distances in kilometers to things out in the open, right? In the open country, right? That's one kilometer. That's two and a half kilometers. But with a little bit of practice, you wouldn't be bad at it. And the same turned out to be true with probabilities, right? So what we do is we give people a series of exercises where they assign probabilities to various statements. And these are at first they're trivia questions. They can be generic trivia questions or specific to their field of expertise. But we might ask questions like, would a hockey puck fit in a golf hole? True or false? And they'd say true. And then I'd say how confident are you? Are you 50% confident like it's a coin flip. You really had no idea. You just pick one randomly, or you're 100% certain you'd say 100%, maybe you're 80 or 90%, confident, etc. They would say how confident they were. We do this With a number of questions over and over again, we'd also ask them questions for a 90% confidence interval. Not to be confused with the term confidence interval that comes up in statistics, Latin is more like a fiducial interval or credible interval. But you you come up with a range that you're 90% sure contains the answer. And we might ask you, when was Napoleon Bonaparte born, what year and you give a range, right? We do this over and over again, we give an initial benchmark test, we find out that people find out that their ranges initially are far too narrow. They don't nearly represent their real uncertainty. We'll get
David Wright:Kahneman's work on Prospect Theory. Right, right. we underestimate tail events, right?
Doug Hubbard:Yeah, well, it's a related concept. Prospect Theory had to do with his empirical analysis of expected utility theory that that Oskar Morgenstern John von Neuman and came up with and theory of games and economic behavior, and a bunch of other people have done some work on that kind of stuff. But he was just doing an empirical analysis of how people actually make risk reward trade offs, as opposed to the theory says, a rational person should
David Wright:I want, I wanted to make that point, because you're about to tell us that it's not actually so universal, aren't you? This problem, because, you know, it's,
Doug Hubbard:it's a which problem, again, the
David Wright:problem of calibrating confidence intervals, right? So you come up with a confidence interval. And so when I walk around my life, right, I read comment, I read whatever, whatever book, you know, relies on that work. And I think, oh, people just can't do this. Right? It's just the fact of human psychology, we cannot estimate confidence intervals or tail events very properly. We're just bad at this kind of thing. But you taught me something different, though.
Doug Hubbard:Oh, yeah. Well, our research shows that after about a half day of training with these exercises, and then also teaching them a few techniques, not just repetition, but a few other techniques, that about 85% of people who go through the training are statistically indistinguishable from a bookie at the end of the day. Amazing. Good, yeah, putting odds on things when you look at all the times they said something was 80%. Likely, it happened about 80% of the time. Yeah, and all the times he said it was 95% likely to happen about 95% of the time, this is what being well calibrated means and of all the times they stated 90% confidence interval for some unknown quantity, like the duration of a project or what their sales will be next quarter, or you know, when Napoleon Bonaparte was born or something, they find out that after training, they can get very close to ranges where about 90% of the data points fall within their stated ranges, they just get better at they don't get better trivia, they get better at quantifying their current state of uncertainty, they just get a better feel for what 80% feels like what 90% feels like etc. Now, here's what's really interesting though, if you have multiple subject matter experts, you talk about getting multiple experts together to agree on things. One of those common methods for getting the estimate out a group of experts is to get them in the room, and they build consensus, and they'll come up with a number. Research shows that this may actually be one of the worst methods coming up with estimates because I mean, obviously the most overconfident people will have a bigger end up having your say and things like that there are methods for aggregating experts that measurably outperform other methods. So if you have a SMI of a subject matter expert, that's the term that gets used a lot. How common that is among your listening. I've
David Wright:heard that before. It's great to me.
Doug Hubbard:So I think that record good. Well, there's algorithms that actually have been studied quite a lot. There's a lot of methods that have been studied over the decades for how you should combine the probability estimates of multiple experts, multiple sneeze. And we've been testing all of these models. And there's been some previous, you know, meta study on this, you know, comparing different models. And we're, we actually have a lot of data because we've, as I said, we've calibrated over 1600 people. And each of those 1600 people answered typically a couple of 100 questions by the time that they're done with their training. So we have many 10s of 1000s of data points on all of these things well over 100,000 total, but we took 434 of the more recent versions of the tests. And of those, that group answered 52,000 questions, total 20,000 true false questions, and 32,000 range questions. And we've been doing this analysis and we're finding out that definitely some methods of aggregation measurably outperform others, we call this the Franken Smee. I don't know what that term. I don't know if I'm going to that term or not. But this is really interesting. If you have two calibrated people that each say independently of each other without communicating if two calibrated people each say that something's 80% likely what percentage of those instances would turn out to be true More than 80%, right? And that's consistent with Bayesian methods. So that's actually consistent. If you can work that out in Bayesian methods, you can think about how you would treat various conditions using Bayes factors, right? And we have this model where we say, what would the Bayesian aggregation of these different conditional probabilities B, how would I add up these conditions that this one experts have this? And this other experts have this? How do I add those up? And we've made this scatterplot on it, and the scatterplot, it actually had an R squared of point nine, eight, coli gees. And so you look at this thing, and you're going, you're saying, Well, you know, here's the theory. And here's what we actually observed in our 52,000 data points. And it's pretty close.
David Wright:So you're telling me that that Bayes theorem and you know, you have a very interesting discussion of Bayes theorem, and I went down a bit of a rabbit hole when I was reading your stuff? I don't know if we can, and we'll see if we can get into it more deeply here. But it's what you're telling me here right now, I think that Bayes theorem actually describes to an R squared of point nine, eight, a feature of human decision making that when you aggregate,
Doug Hubbard:how you should it describes how you should treat how to aggregate the opinions of multiple experts.
David Wright:Yeah, yeah.
Doug Hubbard:And these should, by the way, these will be random pairs of experts answering the same question. These are experts that don't even know each other. They weren't calibrated the same time one could have been calibrated in 2015 and the other in 2018, or something like this. And they both answered the Napoleon Bonaparte question or the hockey puck question or something like this. And one said, I'm 80% sure that the hockey puck will fit in the in the golf hole. And the other said, I'm 70% Sure. You don't average those, with these accurate ends up being slightly more than 80%. So there are ways of aggregating experts. And this is something that not only is based Well, in theory, but has a lot of empirical data. And not just the data I'm doing. There's this has been studied to death for quite a while, we do have a lot of data compared to a lot of academics, though. So in many ways, we're sort of reinforcing what they previously seen with their smaller data sets. So we didn't have to invent any of this, really, we're just saying, hey, we've done all this business. Now we're sharing this data with people. So it's completely consistent with Bayes theorem. It's verified empirically. And so I think it behooves people to think in terms of if some subjective methods measurably outperform other subjective methods, and I feel like I have to have some subjective input in my model, well, then at least I want to use the better subjective methods, right. That's a starting point. And then on top of that, let's just do less of the math in our heads. Let's make the math more explicit. And if we can do that, we can get really good at quantifying our current state of uncertainty about a problem. And that allows us to compute the value of information that was getting circling back to the information, value sort of thing. If you can compute the value of information on all these individual variables, then you can measure what really matters most. And then you don't have to rely so much on the, you know, subject matter expertise, you can conduct empirical measurements, you can do Basie and updates on your prior states of uncertainty, right.
David Wright:I really want to tie this into your framework more deeply like thinking, but because we kind of started in the middle step, which is use experts and calibrate experts, right? So let's say you get, you know, get a particular question. I mean, you almost have this really cool party trick, Doug, where I've seen you do it a few times, and I've read about doing it where somebody was hand you something that's measurable. And you say, Okay, well, let's get to work on this. Right. So like, let's, let's pick one here. So how about know the value of innovation? Right?
Doug Hubbard:Well, I did start doing a webinar called How to measure anything in innovation. So I have some ideas on that particular one. So the first thing we have to ask the first two things we need to ask are, what do you see when you see more of it? Right? So when you see more innovation, what do you actually seen? And just as importantly, why do you care? The Why do you care question helps you frame the problem a little bit better, it helps us figure out what we think we're actually measuring. So why do people want to measure innovation?
David Wright:Oh, because it it well, increases economic growth. It makes profits, right, if you really
Doug Hubbard:think that, but let's think of it in terms of decisions, it's meant to support those are all good things, right. But if I want to measure something, presumably usually in the context that we're talking about, it's because I'm trying to improve some decision. So what decision Am I trying to improve by measuring innovation? I have a few ideas. I don't know if you
David Wright:Yeah, well, I mean, I can go to but I'll try I'll try when when I want to when I want innovate reason why I want innovation. So let's say as a corporate executive, is because I want our company to make more profits. I'm going to build better products. So I want some source of of, of work for people that's valuable for the company. That's what I think of when I think of this.
Doug Hubbard:Oh, Well, let's frame this in terms of a decision then. So the decision might be, which of my teams are more innovative? Right? Sure. Maybe that's the question because I, based on my measure of their innovation, I might assign different teams to different r&d problems.
David Wright:Okay. So I actually just interrupt you again, and you just say, I love it. So this is wonderful. Because, you know, we're another very profound I just want these easy things, it's easy to say, but hard to do, is that, you know, decisions is the mechanism through which actually, you have to think, right, so it's like what you're gonna make when you have this information. It's such a critical piece. And here, I've read your books, and I've listened to him, but I haven't you asked me the question, I still screwed it up. Keep going.
Doug Hubbard:It takes practice, you know, it's not intuitive. Really. It's not complicated. It's not rocket science, but it's not a habit. It's not a habit that people have ingrained right away. So you think in terms of the decision, okay, so the decision is, should I spend more on you know, people that that measure really well on innovation? Because I want to beef up my teams, that's the dilemma I have. Okay. So innovation is a good thing. We all accept whatever it is, we got to define it still. But whatever innovation is we accept, it's a good thing. And so the dilemma might be Should I expend more resources on this? Alright, there's plenty of other decisions you might be able to support. Sometimes people trying to measure the innovation index for a country. Why do you care about that? Well, should I base my r&d there? Can I hire better people in that kind of country? Sure. Can I set up there or something? Or am I using my innovation index of a country? There are indices for this? They're not very good, by the way. But can I use this to forecast something about a country that I can use to inform other decisions? Right, that would be the other thing that I could do. So let's switch gears over to the what do I see when I see more of it? This is the definition problem. This is the What does innovation even mean? Well, um, so Well, before I give you my thoughts, what do you have any ideas on what you see when you see more innovation? What are some observable examples?
David Wright:The solutions to problems that I didn't expect, right? So I, when I see innovation, I think it's creativity, which is another kind of ambiguous term. But I think I handed somebody something new, they're working on something that's hard that I don't understand. And they come up with a solution that I wasn't expecting. So I'm surprised about what they've done.
Doug Hubbard:Sure, right. So that might imply that what you're doing is, let's say you're keeping track of expectations of outcomes initially, and then tracking up preferably adjusted, you know, with some control for bias, right? Like you say, here's what I expect to occur, and somebody other than yourself, or maybe you do it as a blind where you don't even know who the test stars, the teams are. And you say, Okay, in this particular case, I didn't expect the outcome. And there's a few other things, though, I don't think that's enough. I don't think that you know, a Madison Avenue claim is luck. If it doesn't sell. It's not, it's not creative. way to think about it, I wouldn't each make up lots of stuff that's unexpected. We can come up with random stories about things that are unexpected, right? There's some people that are great at Bs, and they can just do this all day, is that innovation? Well, innovation should have other observable outcomes. Like for example, hey, landing a rocket on its tail using propulsive recovery. If, if that's innovative, it should at least work? Well, it does, actually. I mean, maybe, you know, maybe my kids when they were five could have invented the same thing. But there, I just want to work. So that's one thing, it should work. But also, when we say something's innovative, there's a couple of other things that we're thinking of, it tends to imply that we're leading adopters or leading developers as opposed to trailing, right. And it's effective, there's a big benefit in some way it works. And it's effective in some way. There's a lot of value to it in the end. So I may be the leading adopter of something that's of no value at all, is that innovation? Maybe not. I may adopt something that's extremely useful. But I'm not the leading adopter was that innovation, maybe I'm the last hospital to pick up electronic medical records. Is I'm sure they get a lot of benefit out of it, but I wouldn't call that innovation. Okay. So generally, the term innovation I think, people can, you know, debate the definitions here, but I think it generally implies that you're on the early end of something that works that has value to it. You're the early adopter, you're the early developer, okay? And you don't even have to be the first one can We say that customers are innovative, by the way that they adopt things. Like there's some leading customers, there's customers who are leading adopters of new services and technologies. Well, actually, there's a mathematical model called the bass technology diffusion model. It's a technology diffusion model. And it has these two shaped coefficients in it produces the sigmoid curve that kind of tries to model empirically what the adoption rate of a technology in a population is. And it's got these two coefficients. It's got the imitator coefficient and the innovator coefficient. And these two parameters are just too fitting parameters for a curve. The idea being that, if you have a lot of innovators, they tend to adopt things, regardless of who else has adopted them or not. Yep, yeah, if everybody in the population was just an innovator, the growth would be sort of linear looking. People would randomly adopt things, regardless of whether or not other people were adopting them. But if there were some imitators, it would tend to follow this curve that would have an inflection point in it, they would resist adoption at first, and then after you got past some threshold, then it really accelerates because now more people are imitating. And there's been a lot of technologies that have been fit to that kind of models. So it's just a two parameter shaped curve. But that's one use of the term. So one thing we might ask when we're asking about defining new innovation is whose innovation a country's potential employees, maybe Am I maybe I'm asking whether or not a product is innovative. And maybe what people mean by that is, should this be the product I invest in developing versus this other product? Right? They I've heard people use the term innovative that way. In which case, okay, let's figure out what we mean, when we say these things. I ask people, have you seen situations where there's more innovation than other times where there's less innovation? They go? Yeah. Well, what did you see that was different? define it in terms of its observable consequences? Because once you've done that, the rest is trivial math, you're halfway there. Okay. You just have to figure out what's my empirical data collection method? And how do I do the analysis mathematically? Right. So if you're asking the question, I get surprised a lot by their answers. I think that's part of it. Okay. I think it also has to be a valuable answer, right? I think I, whatever area you're in, I think I could probably come up with answers that would surprise you, and would be completely useless. So I don't think that's what you meant by innovation right now, assuming that they made money, and you wouldn't have thought of it otherwise. That's the other part of innovation, I think, is that there's an indication that we wouldn't have thought of it otherwise, that this would be a rare idea that works. It's rare, because we're the early adopters were the early developers of it. And it turns out to be very valuable. So if we think of that, in terms of which r&d project should I invest in, for example, maybe that's what I meant by innovation? Is the product innovative? Okay, well, what am I really doing that? Am I forecasting future sales? So my forecasting something about adoption rate among the population? And which features of products correlate with that when somebody looks at a product and says, I think this product is more innovative than that product? What are they? What do they see when they see more of it? Generally, they define when they come up with examples, they're defining things that they're, they can observe. And they're using that to make forecasts of other observable things. So you've got some input variables that are observations, you have some output variables that you're trying to forecast. And regardless of what model you come up with, you will probably have for most big organizations, you have enough opportunity to test that model over and over again, to keep testing the model. By the way, I'm all about testing models. I think you should be skeptical of miles, applied information economics or anything else, be skeptical of models, that just means test them, you test models, and you adopt things that work. Of course, there's a lot of existing research to build on though you don't have to start brand new from a, you know, a blank sheet of paper, in terms of what actually works. We can build on research. It's already been done. It's been measured before. That's kind of a key concept here, including innovation. When I started putting together the how to measure anything innovation webinar, I started doing my research, just like what I knew the other project, and there's all this really interesting research on does diversity in r&d teams matter? Is there a relationship between the chance that someone's an inventor and their visual spatial IQ, the big that's actually a pretty important one. It's a big visual Not, not other categories of IQ like verbal IQ or something. But visual spatial IQ in particular, turns out to be a pretty good predictor of whether or not someone has a patent. I think that's interesting. All Those things are interesting. And here's another thing that we get as a result of preparing for that webinar. I looked at the Global Innovation index and all which looks at the indices of various, the innovation of various countries. And I look at the corporate innovation, indices that various consulting firms come up with McKenzie and PwC. And also Forbes has an innovation list. What I find interesting is the latter group are completely uncorrelated with each other. If they're measuring something real, and they're measuring something, where more than one of them is, you know, correct, then I should be able to find two that are correlated with each other, and there aren't any. What they do tend to correlate with is both on the countryside and the corporate side is last year's profit or GDP. That's which is a turns out to be a poor predictor of next year's profit or GDP. Oh, apparently, what they're calling innovation is this equation, which uses things like a country's GDP, or corporations growth and profit over an eight quarter period, or however they're defining it. They're using that in their innovation index formula, but that formula is a poor predictor of anything else. So whatever they're measuring, they're either not measuring the same thing, or they're none of them are measuring innovation. Right. So I, I, I'm skeptical of all those indices. I think what people need to do is think in terms of what is it that you're actually forecasting? and for what purpose? And what observations are you making that would support that forecast? You see, people are making these decisions, these estimates intuitively anyway, they're already saying, I use my best judgment, great when you use your judgment, what information is your judgment based on? What experiences is your judgment based on? Because there are no experiences that I can't begin to model is some sort of a historical relationship?
David Wright:Yes, yes. Right.
Doug Hubbard:So you know, if you have experience, you have a data, right? That's all of our experience is is historical data, except with selective recall, and flood inferences. That's, that's what our experience really is. So let's take that experience and quit doing so much of the math in our heads. Let's be more explicit about that. And we can still update our experiences with empirical data, right? We don't have to reject the use of subject matter experts. There's plenty of room for use of subject matter experts and applied information economics, we start with that, actually, we rely on subject matter experts to do things like define the problem. There's no algorithm for that, that we I still have to go to the client say, what's the problem here? What What can you help me understand the thing you're trying to measure? Can you help me understand the decision? subject matter experts are really important for that. They're really important for identifying and quantifying their current state of uncertainty, they always have a current state of uncertainty. That's why you calibrate people. But then after that, you run that value information calculation, and then empirically measure everything else to update their state of uncertainty and make a better bet.
David Wright:So I think you've you've kind of like, quite effectively walked through the the, I think it is the first half, I guess, of your, you know, when approaching a problem and modeling it, there are four assumptions we have to make, right? So one of them is it's been measured before. Another one, you have more data than you think, right? Even if it's at your head, or if it's lying around, you just haven't noticed it next to our you need less data than you think. And there's an important idea there, which you could touch on. And then useful data is actually pretty easy to find. So around like collecting data, you know, phenomenal, phenomenal idea of when you don't know anything about something, even just a little bit of information is incredibly enlightening, right?
Doug Hubbard:Yeah, that's actually true. And that this is, this is another thing that's a surprising result. For some people. For Bayesian analysis methods, you might have run into situations where people assume that because they have a lot of uncertainty, they therefore need a lot of data to measure it, because they have so much uncertainty. But mathematically speaking, just the opposite is true. The more uncertainty you have the bigger uncertainty reduction you get from the first few observations, the way to think of it is if you know almost nothing, almost anything will tell you something. That's, that's more like what the math actually says. So it's in those very cases where we have lots of uncertainty that a little bit of data greatly reduces it and guess which variables have the highest information value. Typically, the very variables that have the highest value to be measured are also for that reason easier to measure. Right? Because we're so uncertain, which is extremely convenient, convenient, you know that the things I really need to measure are actually easier. Measure. You mentioned something before that people choose what what's easy to measure to measure? I'm not really sure that's always true. I think they just measure what they know how to measure, even if the thing they're measuring is very challenging. Like software costs. software development cost is an torturously uncertain and difficult Measurement Problem. Of course, you can measure it just like everything else. But it's hard to measure compared to other things. I think in many cases, benefits of software are actually easier to measure. They're just unfamiliar with the simple methods to do that. Or they're assuming that because they have lots of uncertainty about a productivity improvement, or a future sales increase from updating our customer relationship management system or something like this. They're assuming that because it's so uncertain, they need a lot of data. And in fact, that's not true at all. It they, it's easier to measure because it's uncertain, not in spite of being uncertain
David Wright:I want to tal, we're kind of running low n time. And, man, there's just o much there's so much to cove. So but one issue I really wa t to make sure that we discuss d today is is something that isn t discussed quite explicitly n your books. But I'm fascinat d by as as a subject of enormo s uncertainty. And this is in t e world of innovation again, f r startups, business strateg, right? So here's the proble, right, which is, I want o succeed with a company or I wa t to start a company, right. A d oftentimes, you have th s problem of, nobody's done t before, or you want to launch a new product line, let's say a l these things are sources f innovation. But it's a creati e kind of task, but one whe e usually you'll have kind of o e or two big ideas that you wou d choose to invest in or not, a d you want to rally people arou d it. And I think that, you kno, they say that the mo t successful founders, which, y u know, I think that this mak s sense to me, are those who ki d of are their own customers w o know what the problem is. And o they can, you know, they don t need as much education on i. But have you done much thoug t or spend much time thinki g about business strategy settin, you know, in a more gener l sense, normalcy uncertain, t e frameworks that are availabl, probably fall prey to a lot f the a lot of the criticisms th t we discussed much earlie
Doug Hubbard:Yeah, I mean, I look at say, for example, all of these enterprise risk management or risk management standards out there are really the standards organizations for most things like ISO, NIST at the National Institute for Standards and Technology, the coso standards, each of these, I think they get a lot of fluff in them. Yeah, very fluffy, it's kind of hard to tell what they're really saying. And they make all of these assertions with no empirical data behind it, you know, you know, strategic information falls into three categories. And they come up with three things that sound like kind of, you know, word salad. And they they have no data or even theory, even mathematical theory behind anything that they're saying. I think people should just be a lot more skeptical about all of those methods and really hold them to task because they're, they're affecting things. There's a law, you know, Dodd Frank, that particular set of regulations was talking about the risk management requirements for the corporation, they were referring to the FDIC, the corporation is what they refer to in the law. And they say, right, in the law, the corporation shall use a risk matrix. To evaluate risk. It's It's It's a statement. It's an it's a, it's a demand, but they actually do that. And that's insaan. What is it based on the idea that this is going to help them more realistically assess and then reduce risk? I tell people all the time, your biggest risk, regardless of your industry, is a flawed risk assessment method. If you're if your risk assessment is flawed, if you're going to try to conduct risk management, which is directed in the wrong way, right? If you ask somebody, what do you three biggest risk and the risk assessment method itself is flawed? They don't know what their three biggest risks are, right? It's all this high, medium, low, red, yellow, green stuff that's on a risk matrix. Can I spend $2 million to move this dot from here to here? None of that is actually based on any kind of fundamental theory or math or empirical data of improving things. You really have to be skeptical about
David Wright:why you're there. Do you know like, what, where does this stuff come from? Like, why do people like it?
Doug Hubbard:Well, I think you you kind of hit the nail on the head very early on, you know, what are the objectives of people? If people feel satisfied by the appearance of structured then they are going to be willing to adopt these things and I tell people all the time, astrology is structured. It is astrology is structured and formal. Does it work? No, it has no practical working value at all. But if you want to Feel better by knowing your astrological sign and what you want to do that day? Well, maybe it meets that objective. If that's if you're going to feel better regardless, maybe it meets that objective, okay. But if you actually want to make better decisions in life, I don't think your horoscope is the way to go. I think there's other ways to do that when my wife and I bought our house, or certainly when we started our business and things like this. We ran a big decision model on a Monte Carlo simulation, we did all sorts of analysis on it. We forecasted outcomes, and we make big decisions about how to start things and how to actually progress throughout the years using that method. And of course, now I'm doing much more analysis on my, what my last few years are going to look like before I retire. We're making big decisions based on this stuff. Because I've spent too much time in the research, I really don't trust intuition as much as I used to, or at least unaided intuition. There's lots of intuition you can use, by the way. So we're not rejecting the use of intuition or subject matter expertise. But you want to be skeptical of your own judgment, that's really important. be skeptical of the methods that other people are putting together? Why do they think that works? How do we know this works? That's the question everyone ought to be asking all the time. Even in a very quantitative areas of physics, you know, there's great theoretical foundations for some things, and they still know to ask, how do we know this works? Right? They still need to build a giant particle accelerator to demonstrate the Higgs boson exist, right? So they, they're constantly testing these things. But for some reason, we're not doing that enough in management, consulting, or executive decisions or standards, organizations that just isn't coming up enough. I think it's too easy to generate a lot of stuff. That sounds really good if no one's ever gonna challenge you on it.
David Wright:Yeah. Well, I don't have to stop there, Doug. The two books that I read very, very carefully, where how to measure anything and the failure of risk management. I cannot recommend it highly enough. Doug, where can people find you online? How can they learn more if they're if they're intrigued by this show?
Doug Hubbard:Oh, well, the books have their own website called How to measure anything calm, and my books are listed thre. Our company's website is hubbardresearch.com. And right on there is also the AIE Academy. So if you want to learn more about applied information economics to get into in great detail, the AIE Academy has a whole series of courses, and we have a whole certification process and everything. So we're trying to get these ideas out into the public. We're trying to train more people to do it.
David Wright:And and it rewards deep study, right? This is something you mentioned before, it's not something just sort of snap your fingers and pick up. So I encourage you to do that. Right?
Doug Hubbard:That's true. But I will also say it's a lot easier than learning to become an actuary a lot easier than being an accountant or a lawyer or a doctor. It's not rocket science. Sure. It's complicated enough that not everybody does it simply as a lot of the things that companies do all the time. So
David Wright:Wrongly, we learn. Well, my guest today is Doug Hubbard. Doug, thank you so much for your time today. Really